Decorating for the holidays can include more than putting up a tree and adding tinsel. Decorating can extend to the digital space as well with great Christmas desktop backgrounds and wallpapers.
We’ve collected dozens of great Christmas desktop backgrounds, so you can change your screen every day during the holiday season if you like. While many of these desktop backgrounds and wallpapers are free, others can be found using stock image services or graphic design asset tools or marketplaces. Happy holidays!
Frozen Grunge Christmas Backgrounds
Get into the holiday spirit with this creative frozen grunge Christmas background collection! This unique collection features 10 duotone frozen grunge backgrounds, each in high-resolution JPEG format (5000 x 3500px, 300dpi) perfect for any project.
Frozen Snowflakes Backgrounds
Experience the majestic essence of winter with this Christmas-themed snowflake background collection. This beautifully curated set features ten high-quality, 5000 x 3500px JPEG images, each showcasing a unique snowflake design trapped in icy majesty.
Christmas Bokeh Christmas Backgrounds
A charming collection of Christmas backgrounds with soft-focus winter scenes. This package offers 10 unique designs in a JPEG format, boasting a high resolution of 5000 x 3500px at 300 dpi. Drift into the festive spirit and add a touch of cozy winter sparkle to your projects with this bokeh-inspired set.
Icy Winter Christmas Backgrounds
A captivating array of 10 digitally crafted Christmas backgrounds with icy scenes. It offers a cool, icy take on the typical holiday ambiance with its frozen winter themes. Each high-quality JPEG (5000 x 3500px, 300dpi) transports you to a frosty wonderland, making it a perfect backdrop for various Christmas-themed projects or holiday-inspired aesthetics.
Snowy and Frosty Christmas Backgrounds
A collection of Christmas backgrounds that captures the mystique of winter. Its high-resolution, radiant gradient of frosty textures against a sparkling white snow pattern brings a winter wonderland to life. Versatile and print-ready, this quality backdrop offers pixel dimensions of 3000 x 2000 at 72 dpi.
Magical Christmas Snow Backgrounds
An enchanting set of four high-resolution (4096 x 2304 at 300dpi) Christmas backgrounds, perfect for ushering in the holiday spirit. Each featuring a festive, magical snow design, these backgrounds are versatile and ideal for both web and print media.
3D Christmas and New Years Backgrounds
This is a creative Christmas background that offers a ready-to-use, high-quality, 3D design of a festive scene complete with gift-wrapped boxes under a tree. This 4300 x 2750 px dimension, high-resolution (300 DPI) asset is perfect for enhancing your personal or commercial projects- whether it’s on your website, social media, greeting cards, or promotional materials.
Christmas Trees Backgrounds
A captivating collection of three abstract holiday-themed Christmas backgrounds. These high-quality JPEG files offer a sparkling x-mas tree design in 300 dpi, sized at 3840×2160 pixels. They’re versatile to use, making great additions to your prints, wallpapers, or brochures, bringing a merry touch to your festive season.
Christmas Holiday Backgrounds
This is a delightful collection of four uniquely Christmas background images reflecting the magic and joy of the season. Filled with elements like gifts, fir, snow, gingerbread, and golden decorations, these backgrounds are perfect for adding a festive touch to your website, social media, or blog.
3D Christmas Background with Ornaments
This is a festive, virtual stage to create Christmas backgrounds in a jolly spirit. This 3D asset features a lively, green backdrop adorned with classic Christmas ornaments and balls, and even includes a podium for showcasing your items. It’s the perfect 3D-rendered scene to display your products wrapped in holiday charm.
Free Christmas Backgrounds
Pine & Berries
A beautiful desktop depiction of pine, berries, and mistletoe that still leaves plenty of room for icons on your computer!
Red Christmas
A bold, red Christmas wallpaper that really puts you in the festive mood.
Minimal Christmas Decorations
A beautiful collage of Christmas decorations with a plain background. Stylish and minimal!
Christmas Tree Close-Up Wallpaper
Forget the traditional Christmas tree form, this is a close-up texture-style wallpaper of a holiday classic.
Bringing the Christmas Tree Home
A beautiful tilt-shift image that shows off a Christmas tree on top of a camper van. At a novel scale!
Christmas Garland Desktop
A string of holiday decorations set against a red background.
Star Pattern + Blank Space
A red string and a collection of star decorations, set against a plain dark background.
Single Christmas Ornament
Just a single Christmas ornament, with a stylish depth of field. Wallpaper simplicity!
Snowflake Macro
A super-close-up of one of the most beautiful things in nature — a snowflake. Perfect for your desktop wallpaper.
Mini Christmas Scene
A beautiful little Christmas scene for your desktop wallpaper, with a few Christmas trees and a festive church!
Minimal Snowy Tree
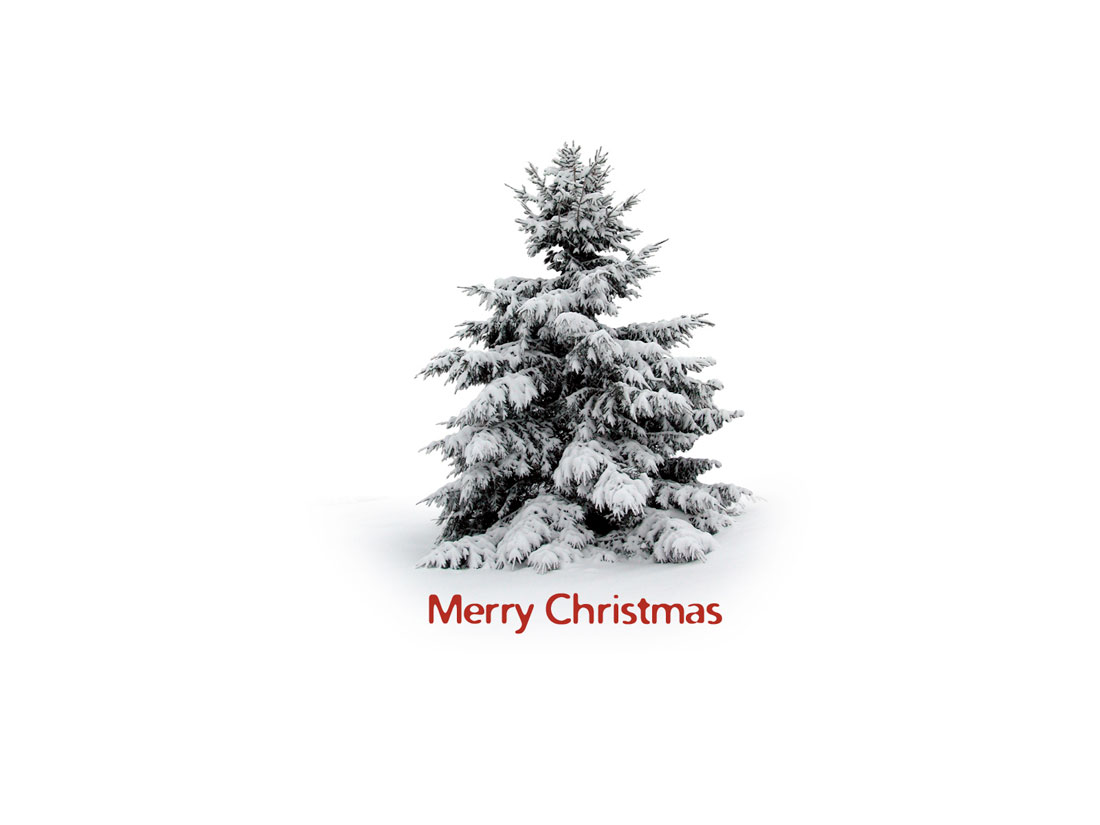
A simple snowy tree – even undecorated – can get you in the holiday spirit. This Christmas desktop background is simple and leaves plenty of room for you to actually see desktop icons.
Santa Flying
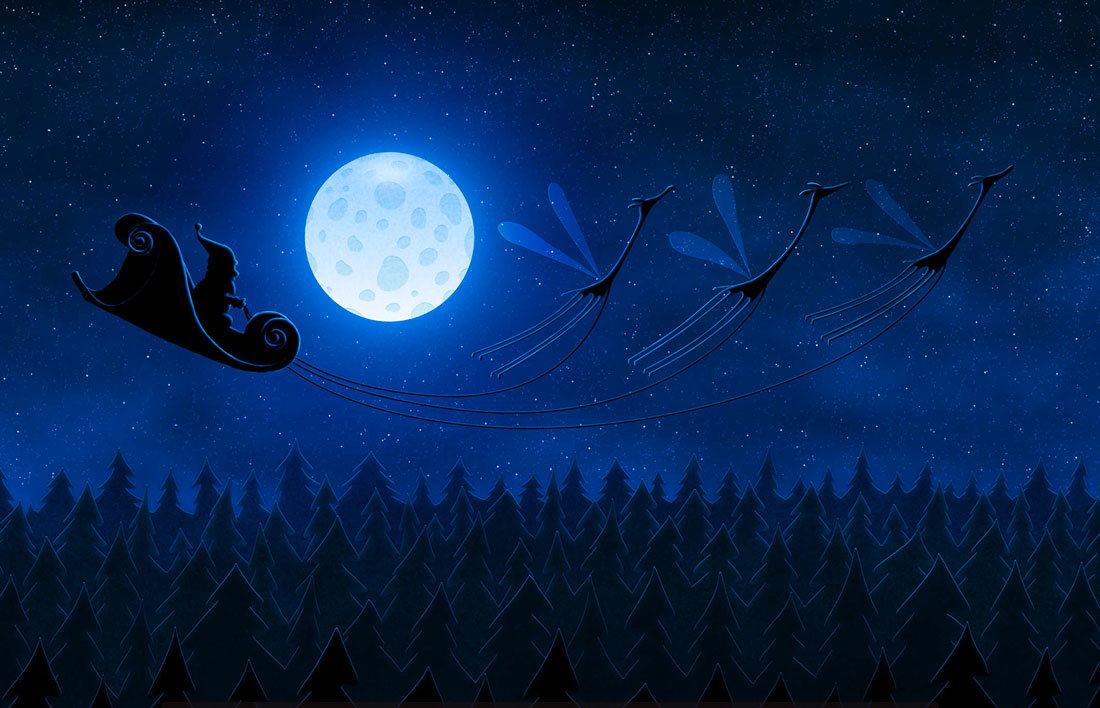
The artistic style of this Santa flying through the sky with his reindeer is a grown-up interpretation of the childhood scene. The dark style is beautiful and not too busy for a desktop wallpaper.
Christmas Tree
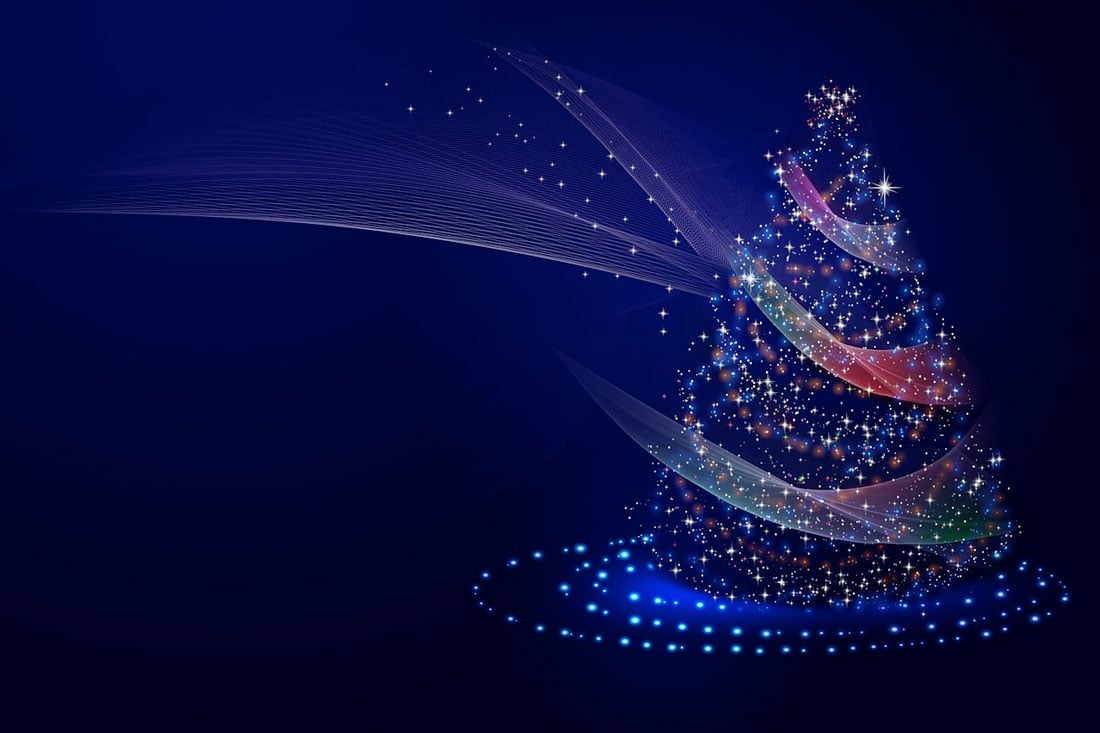
If asymmetrical balance is your style, this Christmas tree wallpaper features a fun style with lights on a dark background.
Blue Christmas
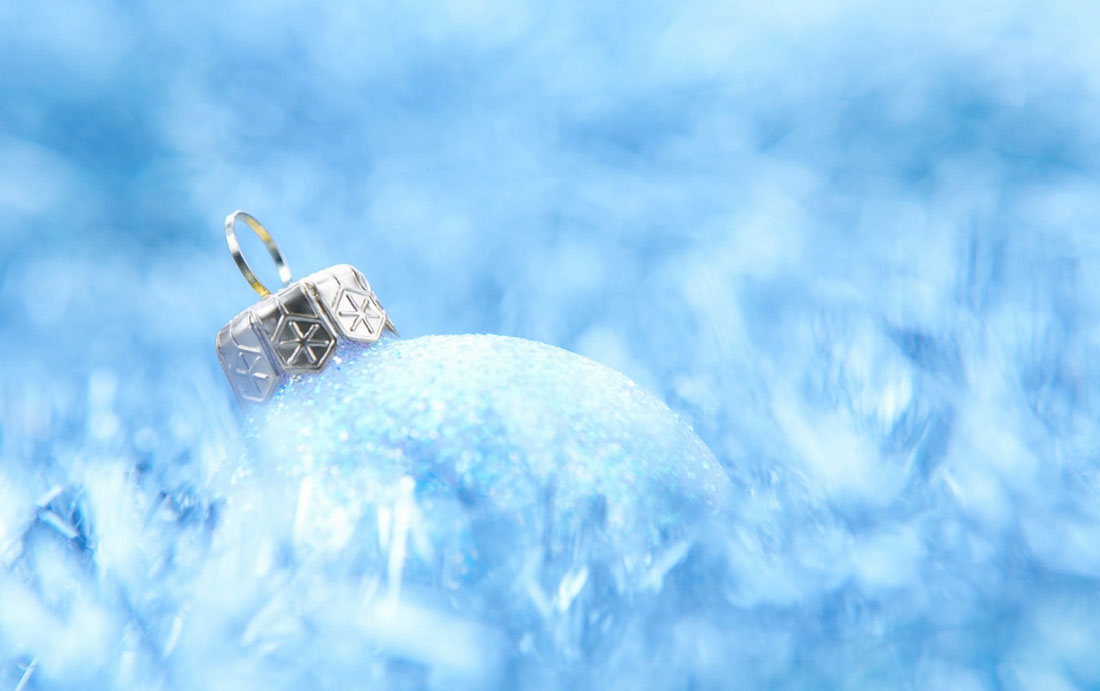
Not every Christmas decoration has to be red and green. This simple blue holiday ornament emanates holiday sparkle.
Gift Delivery
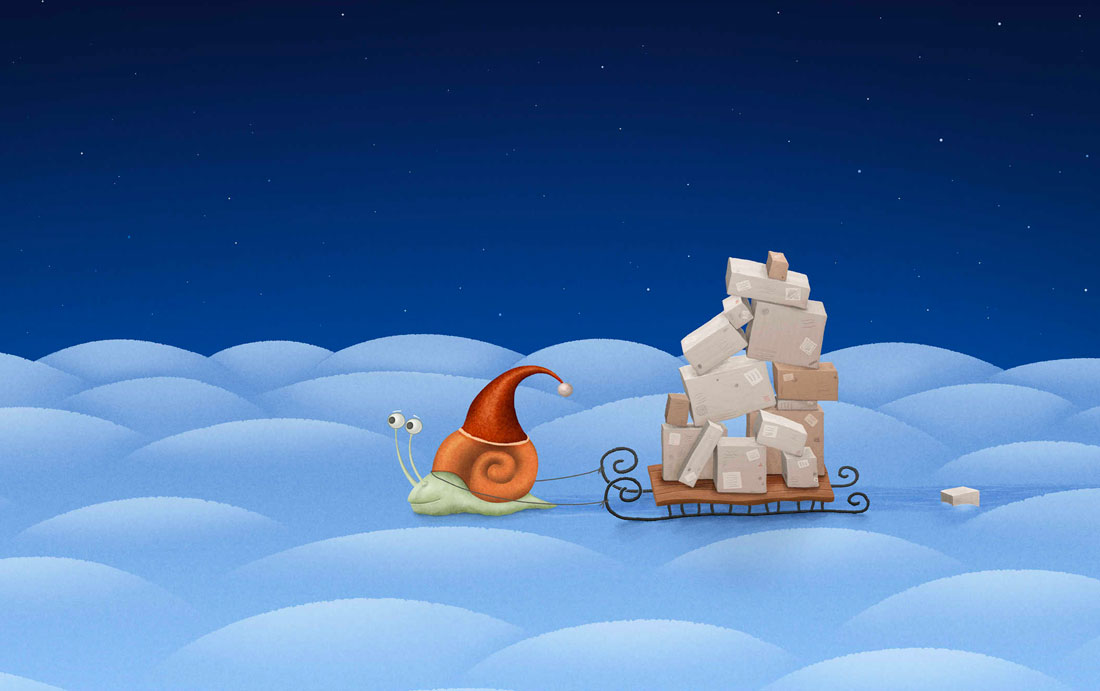
Gift Delivery is a fun representation of how much time it seems to take for the holiday season to arrive. The desktop wallpaper is sure to be a conversation starts if anyone glimpses your screen.
Christmas Letters
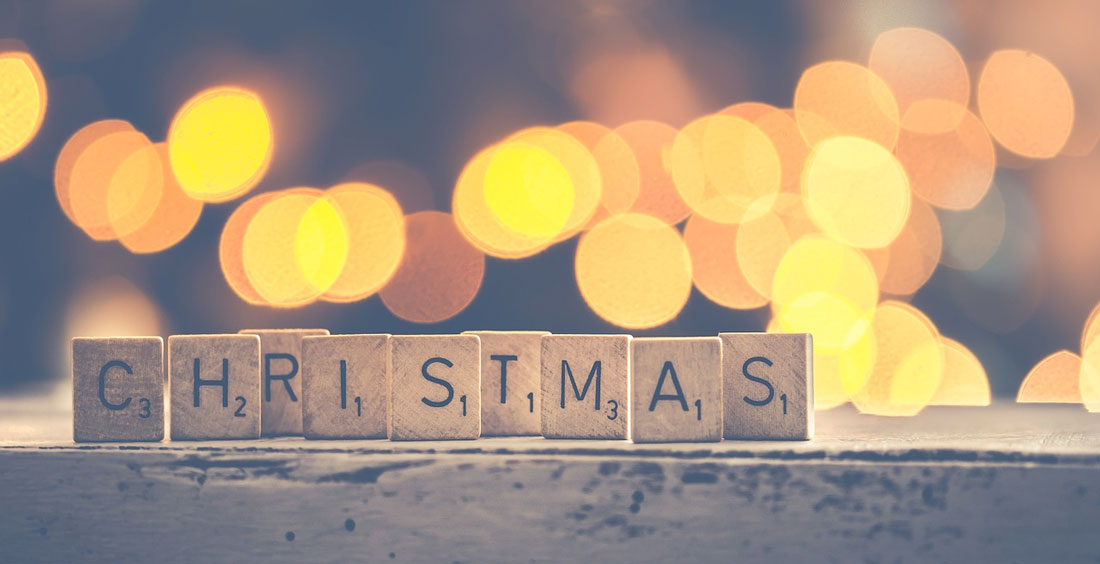
This Christmas desktop background should appeal to all the wordsmiths out there.
Snowman Wallpaper
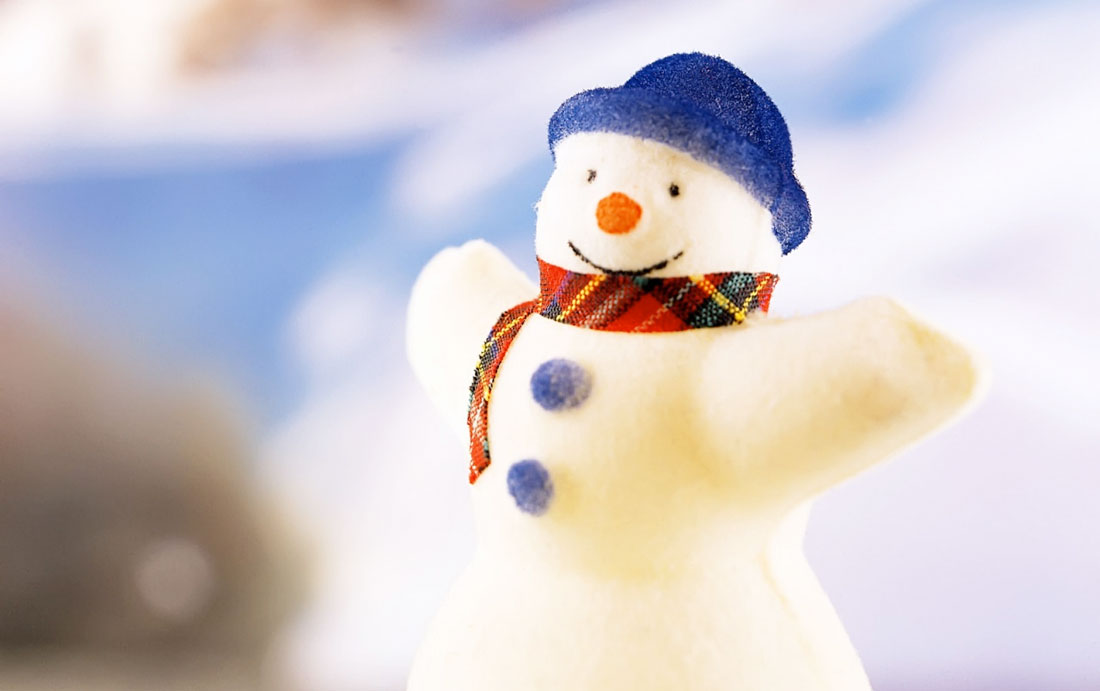
Not every Christmas desktop background has to be just for the holiday, a fun winter scene – such as this lifelike snowman – can be equally nice.
Christmas Garland
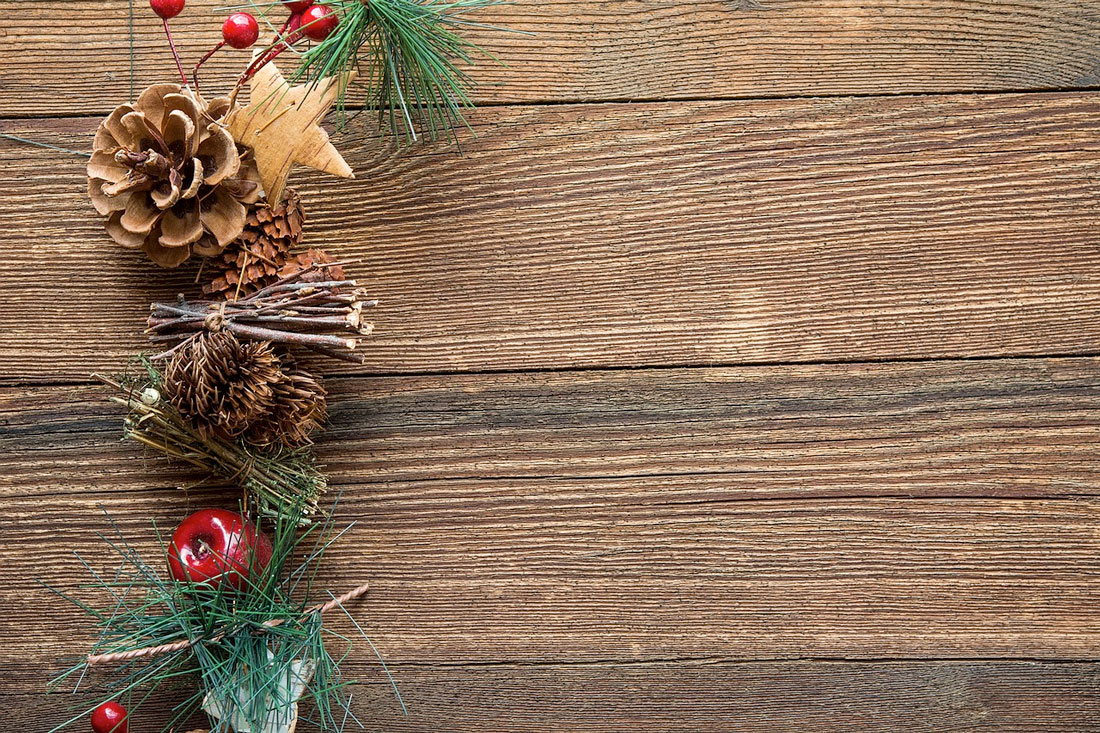
Simple garland on a wood background is eye-appealing and features just a hint of the holiday season for your desktop wallpaper.
Snowman
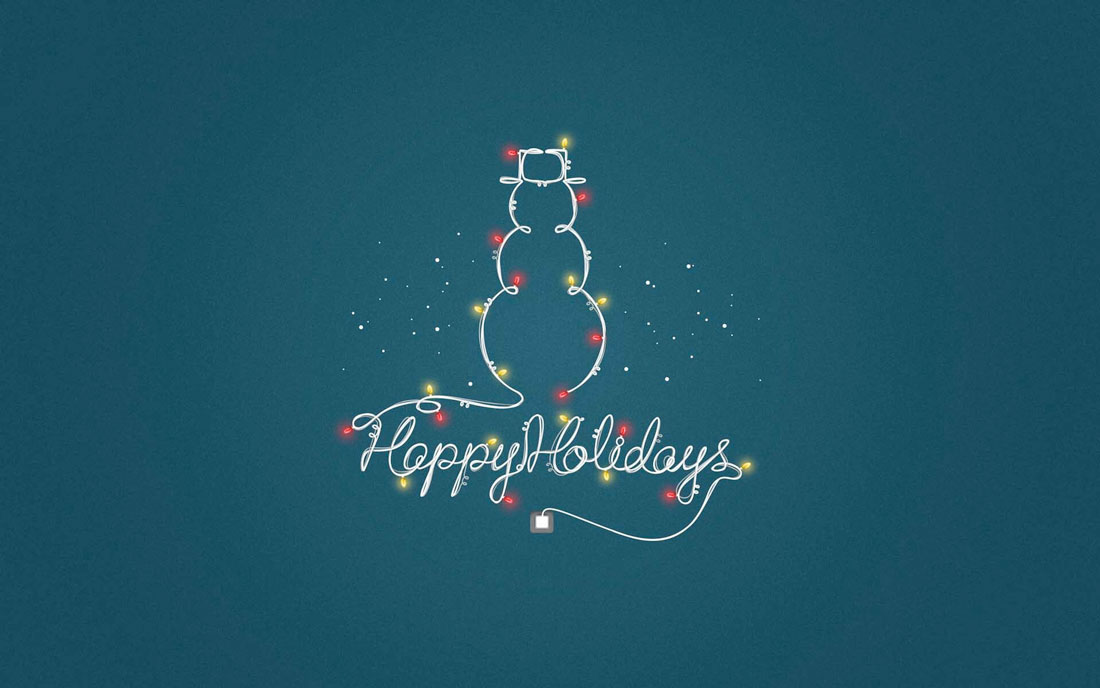
This cool snowman desktop wallpaper features a fun design with plenty of space around it. Great for people that prefer a background without too much going on.
Christmas Volcano
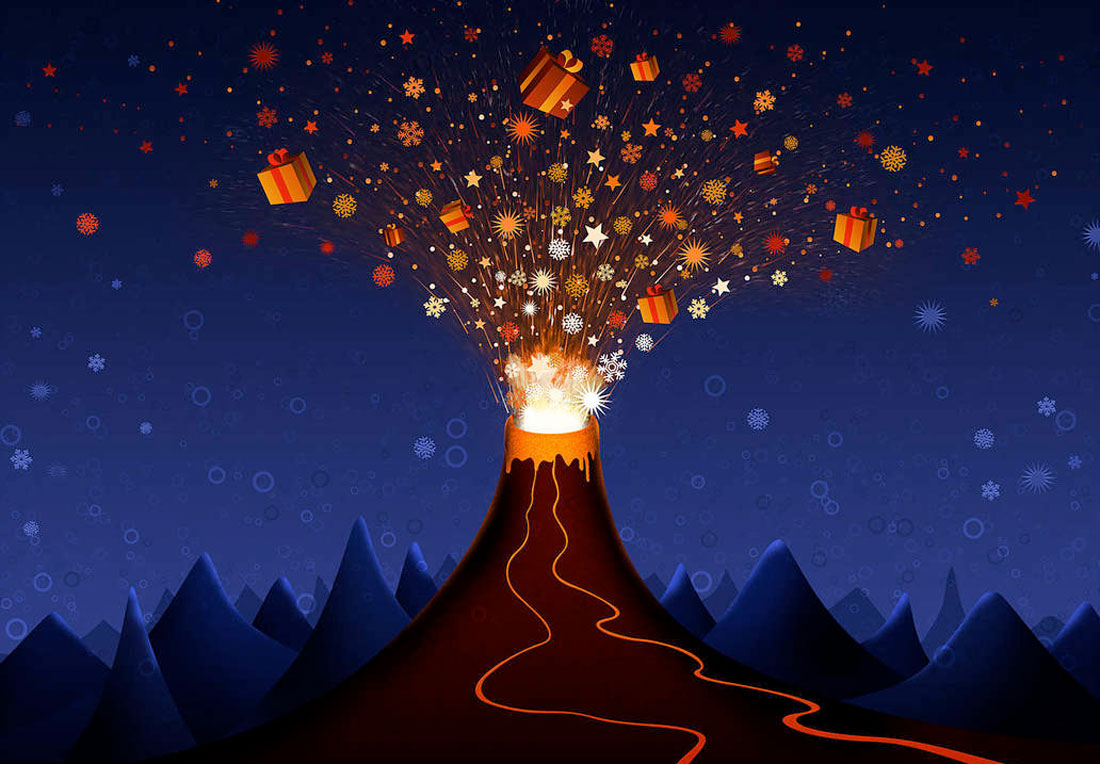
An explosion of gifts is every child’s dream. This representation shows exactly that.
Happy Holidays Sign
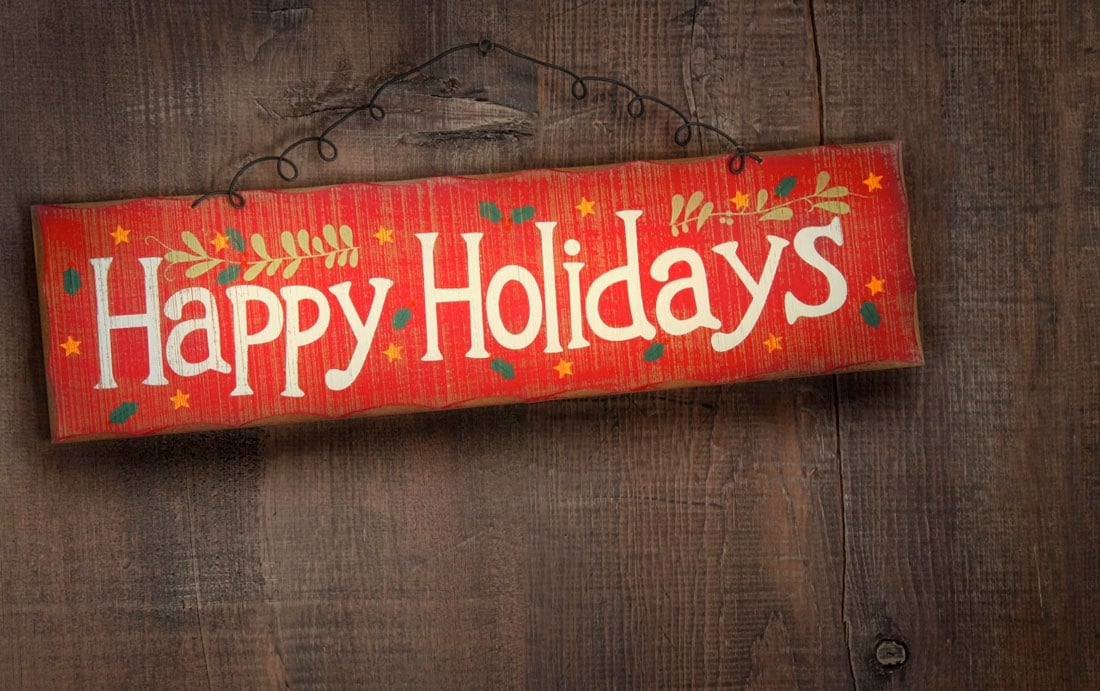
The texture of this Christmas wall paper is nice and provides plenty of room for visible icons on the screen. It has a bit of a trendy and retro vibe.
Christmas Lights
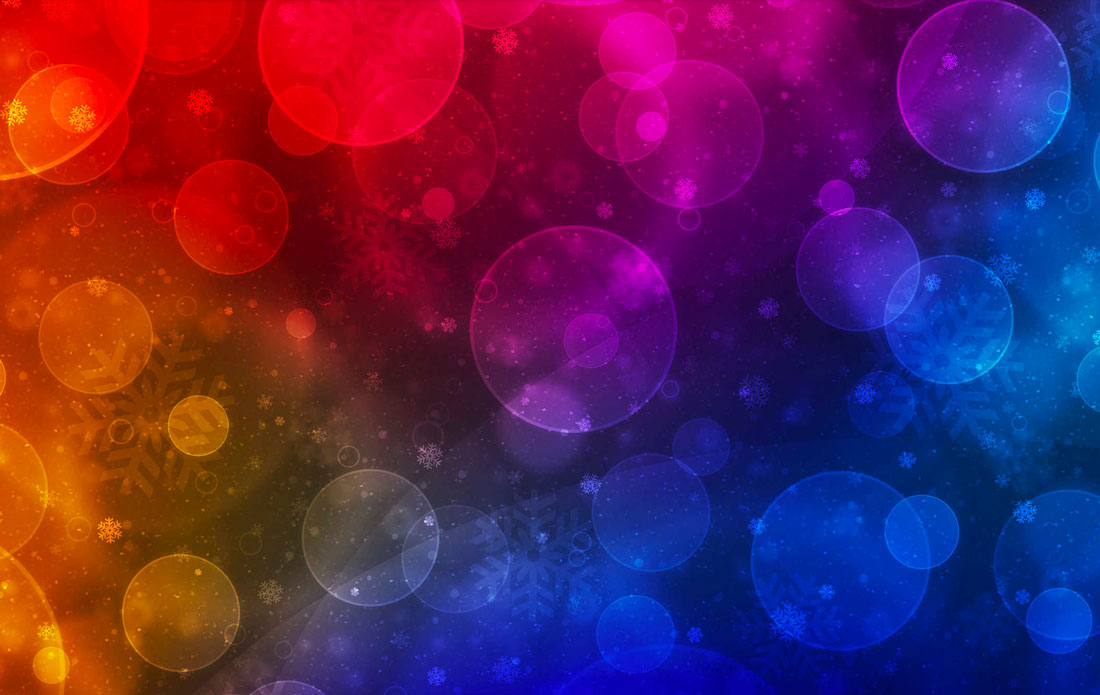
Move a design trend to a holiday trend. This gradient Christmas desktop wallpaper includes fun color, holiday snowflakes and a cheerful design.
Holiday Glitter
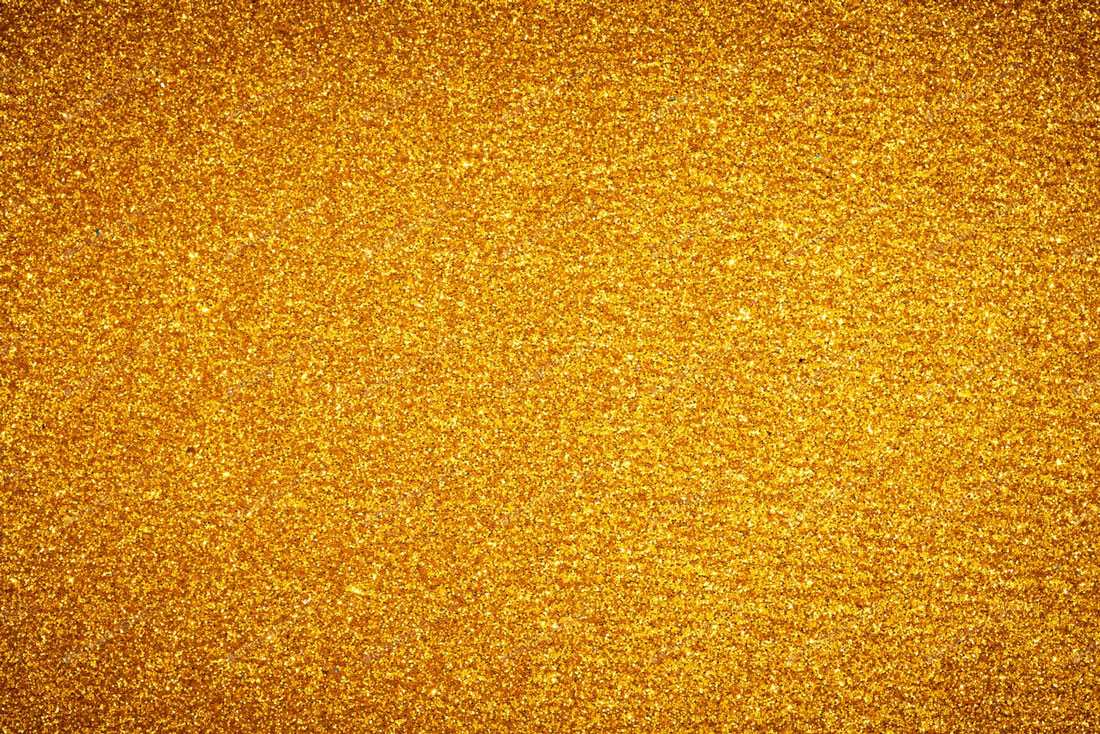
A characteristic of the season is certainly sparkle. This glitter desktop background fits the bill.
Happy New Year
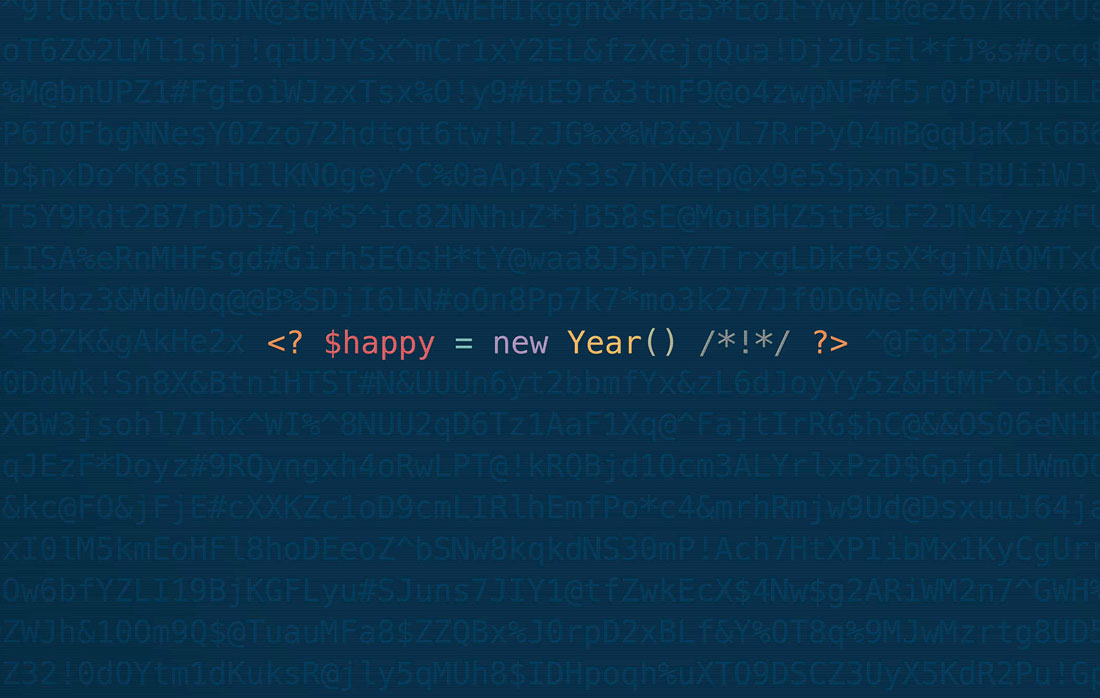
Here’s a little holiday greeting for the devs. Happy New Year is a fun desktop wallpaper to end the holiday season.