Artificial Intelligence (AI) has become an integral part of our lives, from virtual assistants like Siri and Alexa to self-driving cars and advanced recommendation systems.
But how do you measure artificial intelligence performance? How can you determine if they are accurate, efficient, and reliable? In this article, we will explore the basics of measuring AI performance and the challenges and future trends in this field.
- Essential performance indicators for AI
- Difficulties in measuring AI effectiveness
- The future of AI evaluation methods
Measuring AI Systems Effectiveness: Key Performance Metrics
Measuring AI performance involves evaluating how effectively an AI system meets its objectives. Key metrics and methods include:
- Accuracy: This measures the proportion of correct predictions or classifications made by the AI system compared to the total number of predictions. For example, in a classification task, accuracy is calculated as the number of correct predictions divided by the total number of predictions.
- Precision and Recall: Precision indicates the proportion of true positive results among all positive predictions, while recall measures the proportion of true positives among all actual positives. Both metrics are crucial for tasks like object detection or medical diagnosis.
- F1 Score: The F1 score is the harmonic mean of precision and recall, providing a single metric that balances both aspects. It is particularly useful when dealing with imbalanced datasets.
- AUC-ROC: The Area Under the Receiver Operating Characteristic Curve (AUC-ROC) assesses the performance of a binary classification model. It evaluates how well the model distinguishes between classes, with a higher AUC indicating better performance.
- Confusion Matrix: This matrix provides a detailed breakdown of the model’s performance, showing true positives, false positives, true negatives, and false negatives. It helps in understanding where the model is making errors.
- Mean Squared Error (MSE): For regression tasks, MSE measures the average squared difference between predicted and actual values. Lower MSE indicates better model performance.
- Cost-Benefit Analysis: Evaluate the AI system’s performance based on its impact on business outcomes, such as cost savings, increased revenue, or improved customer satisfaction.
Understanding the nuances of these evaluation techniques is essential for accurately gauging AI performance.
The Importance of Measuring AI Performance
Measuring AI performance is crucial for several reasons. Firstly, it allows us to assess the accuracy and efficiency of AI systems. By measuring their performance, we can determine if they are making accurate predictions or decisions and if they are doing so in a timely manner.
This is especially important in applications where accuracy and efficiency are critical, such as healthcare diagnosis or autonomous driving.
Moreover, measuring AI performance enables organizations to make informed decisions about resource allocation and system improvements. By analyzing performance metrics, companies can identify areas for enhancement, whether through additional training data, algorithm adjustments, or hardware upgrades.
This iterative process of measurement and optimization is essential for ensuring that AI systems continue to meet the evolving needs of users and stakeholders.
Ensuring Accuracy and Efficiency
To ensure the accuracy of AI systems, performance metrics are used to evaluate their performance against ground truth data or human expert judgments. These metrics provide quantitative measures of the system’s performance, such as:
- Precision
- Recall,
- F1 score
Similarly, efficiency metrics, such as response time or computational resources required, help assess the system’s efficiency.
The Role of Performance Metrics in AI Development
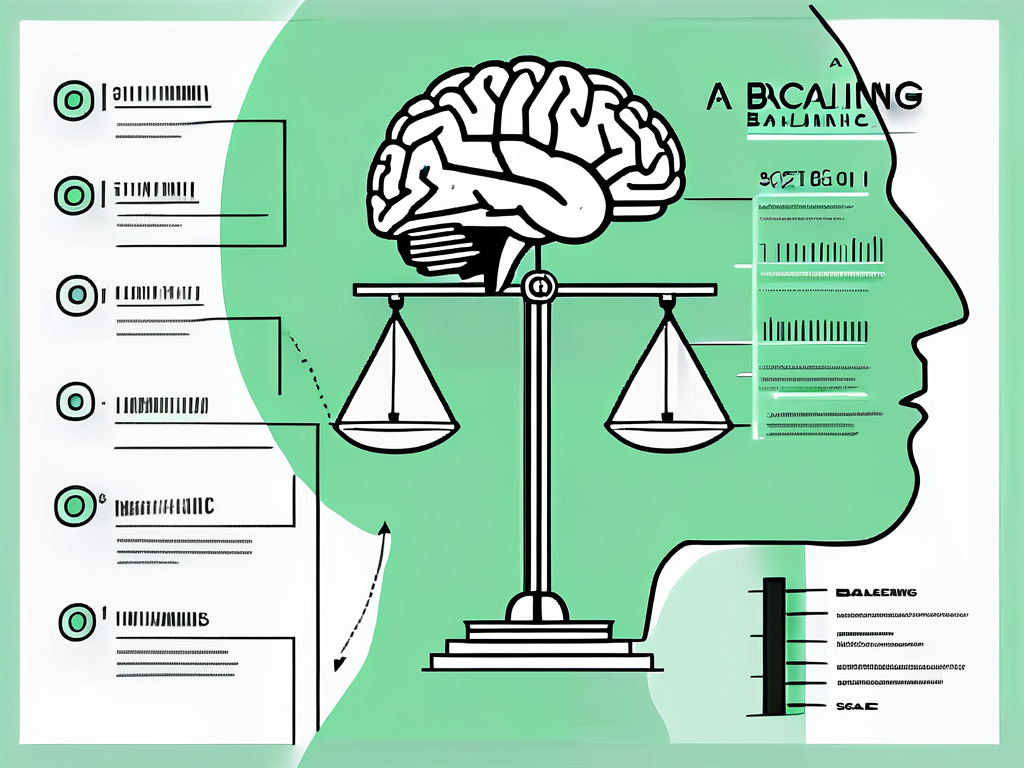
Performance metrics play a vital role in the development of AI systems. They help researchers and developers identify the strengths and weaknesses of their models and algorithms. By measuring performance, they can iteratively improve their models, fine-tune parameters, and optimize algorithms to achieve better accuracy and efficiency.
Key benefits of using performance metrics include:
- Identifying Strengths and Weaknesses: Metrics highlight areas where models perform well and areas needing improvement.
- Iterative Improvement: Continuous measurement allows for fine-tuning and optimization, leading to better model performance.
Performance metrics also serve as benchmarks for comparing different AI approaches and techniques. Researchers can use these metrics to:
- Evaluate State-of-the-Art Methods: Compare various techniques to determine the most effective ones.
- Drive Innovation: Benchmarking against the best practices encourages the development of new and improved methods.
By establishing standard evaluation practices based on performance metrics, the AI community can foster collaboration and knowledge sharing, leading to advancements in AI technology.
Challenges in Measuring AI Performance
Measuring AI performance comes with its challenges. Here are some of the key challenges researchers and developers face:
Complex and Dynamic Environments
AI systems are often deployed in complex and dynamic environments. For example, a self-driving car operates in a constantly changing environment with unpredictable events and road conditions. Measuring the performance of AI systems in such environments is challenging due to high variability and uncertainty.
Key challenges include:
- High Variability: Constantly changing conditions make it difficult to maintain consistent performance metrics.
- Nuanced Evaluations: Traditional performance metrics may not fully capture the complexities of real-world scenarios. For instance, an AI system’s ability to navigate through heavy traffic while ensuring pedestrian safety and adherence to traffic rules adds layers of intricacy to performance evaluation.
The Issue of Bias in AI Performance Measurement
Bias is another significant challenge in measuring AI performance. AI systems learn from data, and if the training data is biased, the system’s performance may also be biased. Bias can lead to inaccurate predictions or decisions, especially if the biases in the training data reflect societal biases or prejudices.
Addressing bias involves:
- Understanding Data Sources: A deep understanding of the data sources and algorithms used in training is crucial.
- Monitoring and Mitigation: Constantly monitor and mitigate biases that may arise during development and deployment stages to ensure fair and accurate performance evaluation.
- Ensuring Fairness: Implement strategies to detect and correct biases, ensuring the AI system makes unbiased predictions and decisions.
By recognizing and addressing these challenges, researchers and developers can improve the accuracy and fairness of AI performance measurements.
Future Trends in AI Performance Measurement
The field of measuring AI performance is constantly evolving, driven by advances in AI technology. Let’s explore some future trends that are shaping this field.
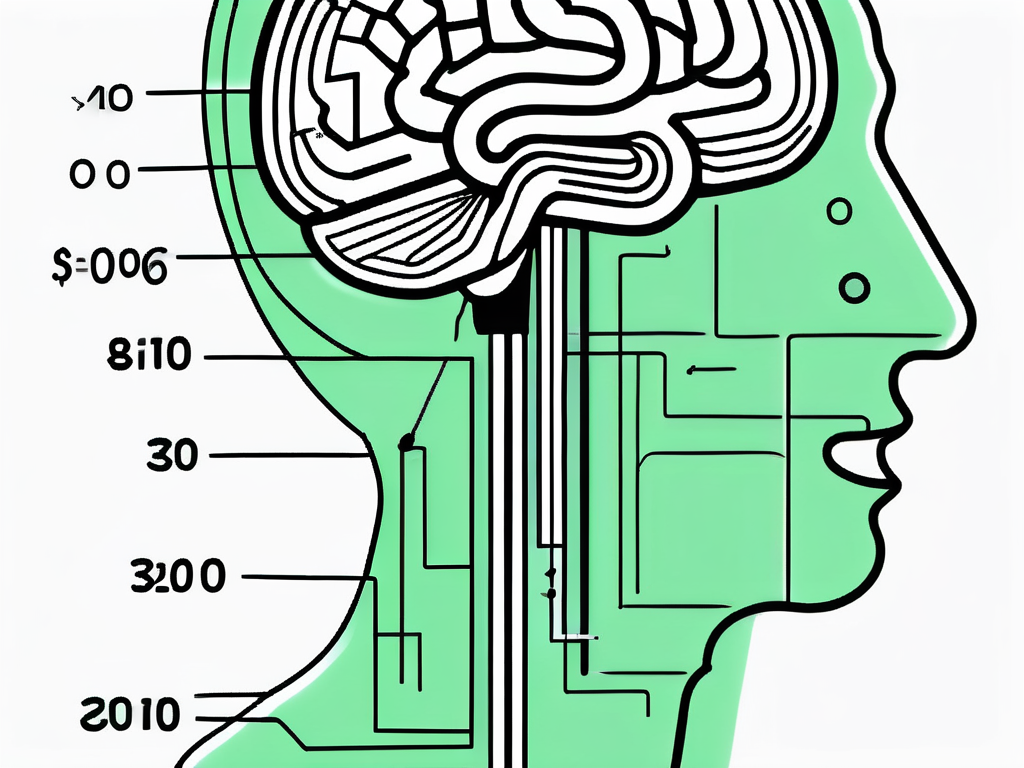
Advancements in AI technology have significantly impacted AI performance measurement. These innovations enable the creation of more accurate, efficient, and reliable AI systems, pushing the boundaries of what can be measured and achieved.
Key impacts include:
- Powerful Machine Learning Algorithms: These allow AI systems to process and analyze vast amounts of data with greater speed and accuracy, improving overall performance and expanding possibilities for measuring capabilities.
- Deep Learning Architectures: These enable AI systems to learn and adapt from complex and unstructured data, enhancing task performance and making performance measurement more nuanced.
- Hardware Accelerators: Improved hardware accelerators boost computational power, enabling faster and more efficient AI processing.
Predictions for AI Performance Metrics
In the future, we can expect the development of new performance metrics that better capture the nuances and complexities of AI systems. They will allow for more comprehensive and responsible evaluation of AI performance.
Key future trends include:
- Fairness Measures: As AI systems become more integrated into various aspects of society, ensuring fairness in decision-making processes becomes crucial. Performance metrics that account for fairness can help identify and address biases or discriminatory outcomes, promoting ethical and responsible AI development.
- Transparency Metrics: AI systems often operate as black boxes, making it difficult to understand how they arrive at their decisions. Metrics that measure transparency can provide insights into AI decision-making processes, allowing for better evaluation and improvement of performance.
- Robustness Measures: Future metrics may also focus on the robustness of AI systems, ensuring they can perform reliably across diverse and challenging conditions.
By embracing these advancements and developing comprehensive performance metrics, we can ensure the responsible and effective deployment of AI technologies.
Driving Innovation with Comprehensive AI Evaluation Techniques
Measuring AI performance is a crucial aspect of developing and deploying AI systems. It allows us to assess their accuracy, efficiency, and reliability.
With the advancements in AI technology and the development of new performance metrics, we can expect even more precise and comprehensive ways to measure AI performance in the future. As AI continues to shape our world, the ability to measure its performance accurately becomes more important than ever.
Measure AI Performance
Hungry for more insights on evaluating AI performance? Explore these articles to enhance your assessment strategies:
These resources will help you refine your approach and ensure your AI systems are performing optimally.